(DOWNLOAD) "Biomarker Analysis in Clinical Trials with R" by Nusrat Rabbee ~ eBook PDF Kindle ePub Free
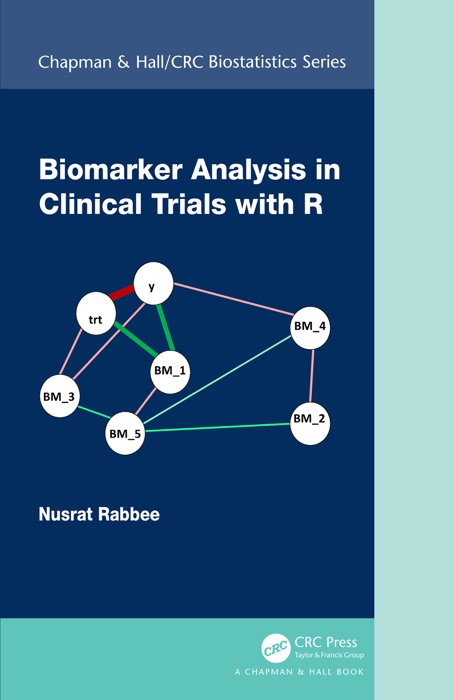
eBook details
- Title: Biomarker Analysis in Clinical Trials with R
- Author : Nusrat Rabbee
- Release Date : January 11, 2020
- Genre: Mathematics,Books,Science & Nature,Professional & Technical,Medical,Pharmacology & Toxicology,Reference,
- Pages : * pages
- Size : 8354 KB
Description
The world is awash in data. This volume of data will continue to increase. In the pharmaceutical industry, much of this data explosion has happened around biomarker data. Great statisticians are needed to derive understanding from these data. This book will guide you as you begin the journey into communicating, understanding and synthesizing biomarker data. -From the Foreword, Jared Christensen, Vice President, Biostatistics Early Clinical Development, Pfizer, Inc.
Biomarker Analysis in Clinical Trials with R offers practical guidance to statisticians in the pharmaceutical industry on how to incorporate biomarker data analysis in clinical trial studies. The book discusses the appropriate statistical methods for evaluating pharmacodynamic, predictive and surrogate biomarkers for delivering increased value in the drug development process. The topic of combining multiple biomarkers to predict drug response using machine learning is covered. Featuring copious reproducible code and examples in R, the book helps students, researchers and biostatisticians get started in tackling the hard problems of designing and analyzing trials with biomarkers.
Features:
Analysis of pharmacodynamic biomarkers for lending evidence target modulation.
Design and analysis of trials with a predictive biomarker.
Framework for analyzing surrogate biomarkers.
Methods for combining multiple biomarkers to predict treatment response.
Offers a biomarker statistical analysis plan.
R code, data and models are given for each part: including regression models for survival and longitudinal data, as well as statistical learning models, such as graphical models and penalized regression models.